招待講演
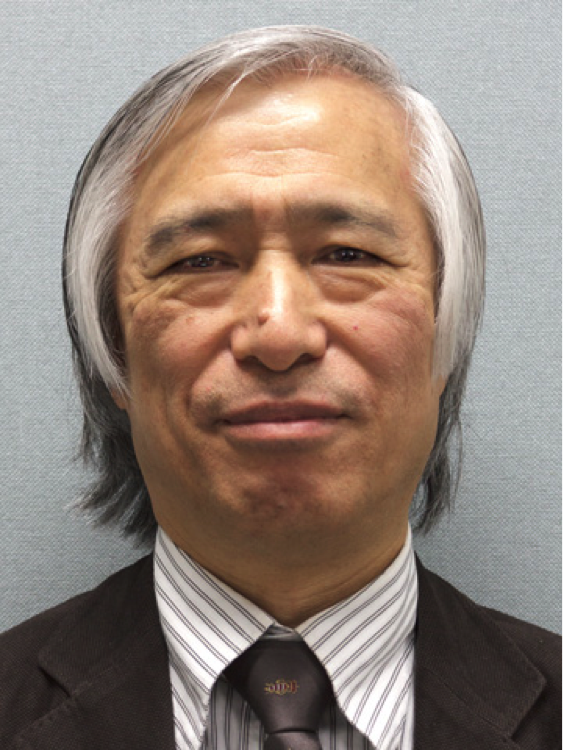
田中 譲
北海道大学 大学院情報科学研究科特任教授
本講演では講演者が参画してきたEUのFP6とFP7におけるガン臨床治験(日本の臨床試験)統合IT支援の研究開発に関する2つのプロジェクト、ACGTとp-medicineの目標と研究開発内容を紹介し、研究開発を担当してきた可視化分析統合環境TOB(Trial Outline Builder)を紹介する。テイラーメイド医療の発見に繋がる分析シナリオは、ワークフロー記述可能な定石的分析シナリオではなく、探索的、試行錯誤的な分析にならざるを得ない。講演者等は、仮説設定に対応するデータ・セグメンテーションと、仮説検証に相当する分析と結果の可視化を、種々の分析ツールを自在に適用して、多様な観点から可視化し、これらの繰り返しの過程を支援する探索的可視化分析が必要と考え、TOBを開発してきた。TOBは臨床治験のマスター・プランのフロー作成と各イベントの入力フォーム定義を作図支援のように支援し、結果をデータベース・スキーマの自動定義に反映する。個々の患者の治療においては、選ばれた分岐に従って、各イベントのフォーム入力がガイドされる。治験終了後は同じグラフィカル表現の環境を用いて、多数のパラメータに関する制約条件を自在に変えながらデータ・セグメンテーションを行い、候補治療法の一つが有意に他より優れた生存率を示すようにケースを絞り込む作業を支援し、テイラーメイド医療の発見を支援する。
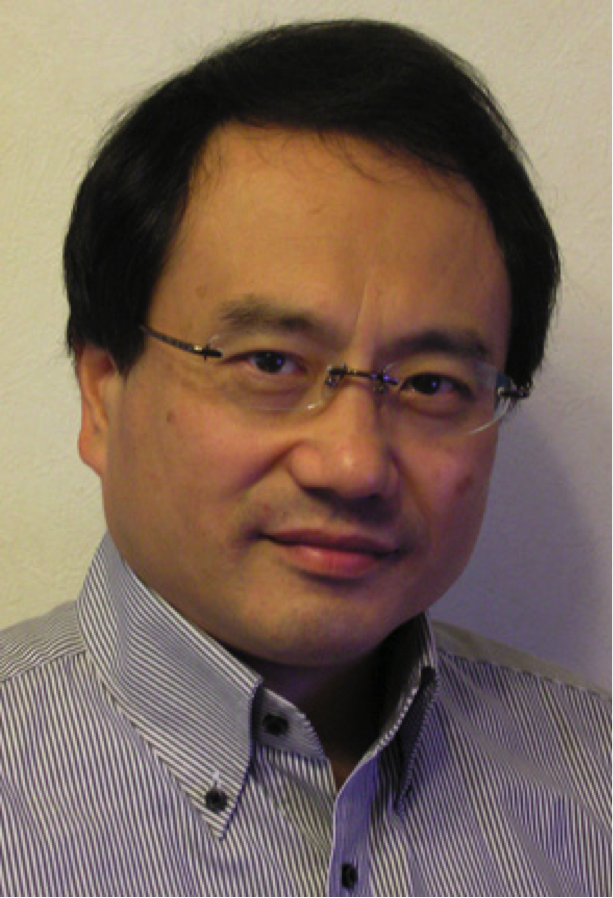
森下真一
東京大学 大学院新領域創成科学研究科 教授
2011年末より Pacific Biosciences 社の1分子リアルタイムDNA解読の可能性をいろいろと探ってきた。DNAポリメラーゼを使った解読であり、平均長で8000塩基、N50 値は10000塩基を超える長いDNA断片配列を解読できる。GC率100%の長い領域も難なく解読できる。一方、高いエラー率(平均15%) に失望する人が多い。我々はIllumina の短いリードを使い修正してみたところ、殆どのエラーは除去できた。個人ゲノムを解読しエラー除去し、標準ゲノム hg19と比較すると、一致率は99.86% となり、残りの0.14% の殆どはこの個人が持つ変異であった。
本当に難しい問題は他にある。(1) エラー修正時に巨大な主記憶を使わずに計算の中間結果を2次記憶に蓄えながら IOボトルネックを避ける工夫 (2) アレル間の違いを示す1塩基の違いをエラー修正で潰さず残しhaplo-typingに活用すること (3) ヘテロ接合の長い挿入や short tandem repeat を検出すること、等の課題の解決に多くの時間を費やした。結果、過去の技術 (short read sequencing, aCGH, fosmid cloning) では不可能であった中規模 (1-10 kb) 構造変化を網羅的に調べることができた。
1分子リアルタイムDNA解読のもう1つの特長は、DNAポリメラーゼが1塩基を解読する時間の遅延としてDNA修飾を「直接」検出する能力である。2009年頃から研究され、微生物DNAの多様な修飾を検出するため普及している。しかし脊椎動物、特に哺乳類で注目されるCpG のメチル化の場合、解読時間の遅延は微小で観測は困難であった。我々は周辺のCpG のメチル化状態を考慮すると検出精度が非常に高くなることに注目し、線形時間アルゴリズムを設計した。結果、従来は測定困難だったDNA重複領域やLINE1等のリピート配列上のDNAメチル化状態を網羅的に調べられるようになった。1分子リアルタイムDNA解読の応用可能性はひろい。講演では今後の可能性についても紹介したい。
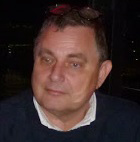
Vladimir Brusic
(Professor, Cancer Vaccine Center, Dana-Farber Cancer Institute, Boston, USA)
The World’s total data is doubling every two years where quantity, complexity, and types of data are rapidly expanding. The enormous rate of generation and digital access to data is profoundly changing the way how business is conducted. Biomedical data include pharmaceutical R&D data, clinical data, activity and cost data, patient behavior data, basic science data, standards and ontologies, among others. Furthermore, Big Data approaches are increasingly needed for utilization of results from various Omics studies. Specific applications include predictive and content analytics that support drug discovery and optimization, the development of new diagnostic methods, and personalization of medicine. Biomedical data vary in granularity, quality, and complexity. There is a variety of sources and data formats – web pages, publications, technical reports, and databases. The challenge is to make the transition from data to actionable knowledge. We propose the use of knowledge-based approaches whereby well-annotated data are combined with specialized analytical tools and integrated into analytical workflows. A set of well-defined workflow types with rich summarization and visualization capacity facilitates the transformation from data to critical information and knowledge that enable understanding, decision making, and selection of actions for solving various problems. The emerging Big Data requires dynamic integration of standardized data into knowledge bases and also make selected data sources accessible through integration with the analytical tools. We will demonstrate several applications including proteogenomic applications for cancer profiling, proteomics applications for profiling T-cell immunome, and discovery of vaccine targets. In the initial step of Big Data analysis, data must be subject to quality control, error elimination, and filtering. The data is then analyzed and refined for different modeling purposes (eg. summarization, reporting, exploratory analysis, or discovery). The refined data is subsequently added to the data sources. Multi-level mathematical models are also integrated with the knowledgebase to perform simulations and predictions for predictive analytics. The Big Data is a resource that is increasingly important for the improvement of diagnosis, prognosis, and monitoring of the disease, facilitation of selection and optimization of therapies, and the betterment of lifestyle.