Keynote Speakers
In the presentation order.
Masami Yokota HIRAI
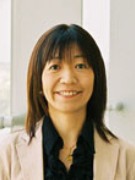
Metabolic Systems Research Unit,
Center for Sustainable Resource Science, RIKEN
Talk title : Understanding of plant metabolism via metabolomics-based mathematical modeling
AbstractOver the past decade, it has become possible to acquire a large metabolome dataset from high-throughput technologies based on mass spectrometry and nuclear magnetic resonance. We consider time-series metabolome data especially important to understand metabolic reaction networks because they include in vivo kinetic information of networks. We are developing a new approach to integrate the time-series metabolome data with metabolic reaction network for building a large-scale kinetic model. In this approach, a mathematical model is constructed using power-law representation within the framework of Biochemical Systems Theory.The model parameters are simultaneously estimated using only actual time-series data of metabolite concentrations. The obtained model enables us to simulate dynamic behaviors of metabolite concentrations and perform system analysis of the network. In this presentation the concept and the results of our on-going study will be introduced.
References:
- Hirai, M.Y., Yano, M., Goodenowe, D.B., Kanaya, S., Kimura, T., Awazuhara, M., Arita, M., Fujiwara, T., and Saito, K. (2004) Integration of transcriptomics and metabolomics for understanding of global responses to nutritional stresses in Arabidopsis thaliana. Proc. Natl. Acad. Sci. U. S. A. 101, 10205-10210.
- Sawada, Y., Akiyama, K., Sakata, A., Kuwahara, A., Otsuki, H., Sakurai, T., Saito, K., and Hirai, M.Y. (2009) Widely targeted metabolomics based on large-scale MS/MS data for elucidating metabolite accumulation patterns in plants. Plant Cell Physiol. 50, 37-47.
- Sriyudthsak, K., Shiraishi, F., and Hirai, M.Y. (2013) Identification of a metabolic reaction network from time-series data of metabolite concentrations. PLoS One 8, e51212.
- Sriyudthsak, K., Iwata, M., Hirai, M.Y., and Shiraishi, F. (2014) PENDISC: a simple method for constructing a mathematical model from time-series data of metabolite concentrations. Bull Math Biol. 76, 1333-1351.
- Sriyudthsak, K., Sawada, Y., Chiba, Y., Yamashita, Y., Kanaya, S., Onouchi, H., Fujiwara, T., Naito, S., Voit, E.O., Shiraishi, F., and Hirai, M.Y. A U–system approach for predicting metabolic behaviors and responses based on an alleged metabolic reaction network. Accepted in GIW2014.
9:00 in the morning, Monday, December 15, 2014
Thomas LENGAUER
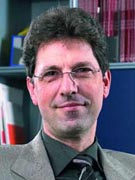
Computational Biology and Applied Algorithmics,
Max-Planck-Institut für Informatik
Talk title : Custom-tailoring combination drug therapies with bioinformatics
AbstractOver the past decade bioinformatics technology has advanced to effectively aid the selection of drug therapy in this setting. Forerunner is the HIV infection leading to AIDS, where bioinformatics-assisted therapy selection has entered clinical routine. Hepatitis C and Hepatitis B are following. Cancer has a similar evolutionary characteristic. Here, the role of the pathogen is taken by the aberrant tumor genome. Research in the cancer field is expected to follow in the tracks of the developments for viral diseases.
We report on the state of the art in the field of bioinformatics-supported resistance analysis and give perspectives of further developments.
Selected publications:
- Lengauer, T., Pfeifer, N. and Kaiser, R. (2014). “Personalized HIV therapy to control drug resistance.” Drug Discovery Today: Technologies 11:57-64.
- Bozek, K., et al. (2013). “Analysis of Physicochemical and Structural Properties Determining HIV-1 Coreceptor Usage.” PLoS Comput Biol 9(3): e1002977.
- Bock, C. and T. Lengauer (2012). “Managing drug resistance in cancer: lessons from HIV therapy.” Nat Rev Cancer 12(7): 494-501.
- Bogojeska, J. and T. Lengauer (2012). “Hierarchical Bayes model for predicting effectiveness of HIV combination therapies.” Stat Appl Genet Mol Biol 11(3): Article 11.
- Pfeifer, N. and T. Lengauer (2012). “Improving HIV coreceptor usage prediction in the clinic using hints from next-generation sequencing data.” Bioinformatics 28(18): i589-i595.
1:00 in the afternoon, Monday, December 15, 2014
Limsoon WONG
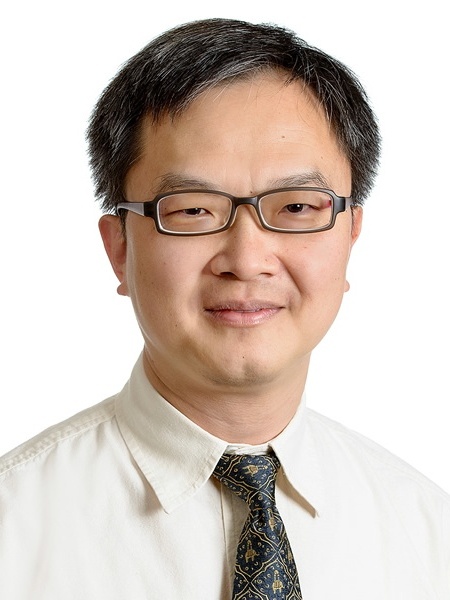
National University of Singapore
Talk title : Delivering a quantum leap in the reproducibility, precision, and sensitivity of gene-expression-profile analysis even when sample size is extremely small
Abstract8:30 in the morning, Tuesday, December 16, 2014
Janet KELSO
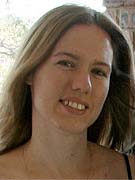
Minerva Research Group for Bioinformatics,
Max Planck Institute for Evolutionary Anthropology
Talk title : What we have learned from sequencing archaic human genomes
AbstractI will outline some of the challenges in the generation and analysis of ancient genome sequence data, and discuss the evolutionary insights that have resulted from the sequencing of these genomes.
References
- K. Prufer, F. Racimo, N. Patterson et al. The complete genome sequence of a Neanderthal from the Altai Mountains. Nature 505, 43-49 (2014).
- M. Meyer, M. Kircher, M. T. Gansauge et al. A high-coverage genome sequence from an archaic Denisovan individual. Science 338, 222-226 (2012).
- S. Sankararaman, S. Mallick, M. Dannemann et al. Nature 507, 354-357 (2014).
1:00 in the afternoon, Tuesday, December 16, 2014
Shinya KURODA
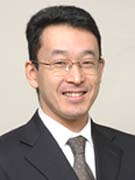
Department of Biophysics and Biochemistry,
Graduate School of Science, The University of Tokyo
Talk title : Temporal coding of insulin action
Abstract9:00 in the morning, Wednesday, December 17, 2014
Alfonso VALENCIA
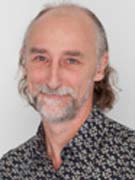
Structural Computational Biology Group,
Spanish National Cancer Research Centre
[homepage] Email : valencia_AT_cnio.es
Talk title : Cancer Genomics and Computational Biology
AbstractReferences
- Valencia A & Hidalgo M (2012) Getting personalized cancer genome analysis into the clinic: the challenges in Bioinformatics. Genome Medicine, 461
- Vazquez M, de la Torre V , Valencia A (2012) Chapter 14: Cancer Genome Analysis, in Translational Bioinformatics PLOS Computational Biology open access book.
- de Juan D, Pazos F, Valencia A. (2013) Emerging methods in protein co-evolution. Nat Rev Genet. 14:249-61.
- Rodriguez JM, Maietta P, Ezkurdia I, Pietrelli A, Wesselink JJ, Lopez G, Valencia A, Tress ML. (2013) APPRIS: annotation of principal and alternative splice isoforms. Nucleic Acids Res. 41:D110-7.
- Ibañez C, Boullosa C, Tabarés-Seisdedos R, Baudot A and Valencia A (2014) Molecular Evidence for the Inverse Comorbidity between Central Nervous System Disorders and Cancers detected by Transcriptomic Meta-analyses. Plos Genet, in press.
4:00 in the afternoon, Wednesday, December 17, 2014